In [1]: index = pd.MultiIndex.from_product([range(3), ['one', 'two']], names=['first', 'second'])
2.14 The query()
Method (Experimental)
New in version 0.13.
DataFrame
objects have a query()
method that allows selection using an expression.
You can get the value of the frame where column b
has values
between the values of columns a
and c
. For example:
In [2]: n = 10
In [3]: df = pd.DataFrame(np.random.rand(n, 3), columns=list('abc'))
In [4]: df
Out[4]:
a b c
0 0.2370 0.0076 0.0198
1 0.3131 0.0995 0.1952
2 0.2073 0.1649 0.7119
3 0.0321 0.1974 0.9646
.. ... ... ...
6 0.8615 0.3707 0.3688
7 0.5429 0.6828 0.8229
8 0.1314 0.0776 0.8409
9 0.2210 0.7529 0.3430
[10 rows x 3 columns]
# pure python
In [5]: df[(df.a < df.b) & (df.b < df.c)]
Out[5]:
a b c
3 0.0321 0.1974 0.9646
4 0.5739 0.6992 0.9746
7 0.5429 0.6828 0.8229
# query
In [6]: df.query('(a < b) & (b < c)')
Out[6]:
a b c
3 0.0321 0.1974 0.9646
4 0.5739 0.6992 0.9746
7 0.5429 0.6828 0.8229
Do the same thing but fall back on a named index if there is no column
with the name a
.
In [7]: df = pd.DataFrame(np.random.randint(n / 2, size=(n, 2)), columns=list('bc'))
In [8]: df.index.name = 'a'
In [9]: df
Out[9]:
b c
a
0 4 2
1 3 2
2 2 0
3 1 3
.. .. ..
6 4 3
7 3 0
8 2 3
9 2 4
[10 rows x 2 columns]
In [10]: df.query('a < b and b < c')
Out[10]:
Empty DataFrame
Columns: [b, c]
Index: []
If instead you don’t want to or cannot name your index, you can use the name
index
in your query expression:
In [11]: df = pd.DataFrame(np.random.randint(n, size=(n, 2)), columns=list('bc'))
In [12]: df
Out[12]:
b c
0 1 8
1 3 3
2 5 1
3 9 2
.. .. ..
6 6 9
7 2 1
8 1 0
9 0 8
[10 rows x 2 columns]
In [13]: df.query('index < b < c')
Out[13]:
b c
0 1 8
Note
If the name of your index overlaps with a column name, the column name is given precedence. For example,
In [14]: df = pd.DataFrame({'a': np.random.randint(5, size=5)})
In [15]: df.index.name = 'a'
In [16]: df.query('a > 2') # uses the column 'a', not the index
Out[16]:
Empty DataFrame
Columns: [a]
Index: []
You can still use the index in a query expression by using the special identifier ‘index’:
In [17]: df.query('index > 2')
Out[17]:
a
a
3 0
4 2
If for some reason you have a column named index
, then you can refer to
the index as ilevel_0
as well, but at this point you should consider
renaming your columns to something less ambiguous.
2.14.1 MultiIndex
query()
Syntax
You can also use the levels of a DataFrame
with a
MultiIndex
as if they were columns in the frame:
In [18]: n = 10
In [19]: colors = np.random.choice(['red', 'green'], size=n)
In [20]: foods = np.random.choice(['eggs', 'ham'], size=n)
In [21]: colors
Out[21]:
array(['green', 'red', 'red', 'green', 'red', 'green', 'green', 'red',
'green', 'red'],
dtype='|S5')
In [22]: foods
Out[22]:
array(['eggs', 'eggs', 'eggs', 'eggs', 'eggs', 'ham', 'eggs', 'eggs',
'eggs', 'eggs'],
dtype='|S4')
In [23]: index = pd.MultiIndex.from_arrays([colors, foods], names=['color', 'food'])
In [24]: df = pd.DataFrame(np.random.randn(n, 2), index=index)
In [25]: df
Out[25]:
0 1
color food
green eggs 0.2513 -0.1437
red eggs 0.1958 -0.8763
eggs -0.7964 0.2935
green eggs -0.5310 1.5091
... ... ...
eggs 0.0068 -0.8226
red eggs 0.3901 0.6442
green eggs -0.7360 -1.0821
red eggs -0.4404 0.0792
[10 rows x 2 columns]
In [26]: df.query('color == "red"')
Out[26]:
0 1
color food
red eggs 0.1958 -0.8763
eggs -0.7964 0.2935
eggs 0.5069 0.5864
eggs 0.3901 0.6442
eggs -0.4404 0.0792
If the levels of the MultiIndex
are unnamed, you can refer to them using
special names:
In [27]: df.index.names = [None, None]
In [28]: df
Out[28]:
0 1
green eggs 0.2513 -0.1437
red eggs 0.1958 -0.8763
eggs -0.7964 0.2935
green eggs -0.5310 1.5091
... ... ...
eggs 0.0068 -0.8226
red eggs 0.3901 0.6442
green eggs -0.7360 -1.0821
red eggs -0.4404 0.0792
[10 rows x 2 columns]
In [29]: df.query('ilevel_0 == "red"')
Out[29]:
0 1
red eggs 0.1958 -0.8763
eggs -0.7964 0.2935
eggs 0.5069 0.5864
eggs 0.3901 0.6442
eggs -0.4404 0.0792
The convention is ilevel_0
, which means “index level 0” for the 0th level
of the index
.
2.14.2 query()
Use Cases
A use case for query()
is when you have a collection of
DataFrame
objects that have a subset of column names (or index
levels/names) in common. You can pass the same query to both frames without
having to specify which frame you’re interested in querying
In [30]: df = pd.DataFrame(np.random.rand(n, 3), columns=list('abc'))
In [31]: df
Out[31]:
a b c
0 0.1427 0.8672 0.1419
1 0.7378 0.7852 0.5929
2 0.1730 0.9451 0.1403
3 0.4987 0.0624 0.7161
.. ... ... ...
6 0.0155 0.7807 0.5830
7 0.8813 0.4309 0.6147
8 0.6297 0.2569 0.3005
9 0.8960 0.7063 0.2833
[10 rows x 3 columns]
In [32]: df2 = pd.DataFrame(np.random.rand(n + 2, 3), columns=df.columns)
In [33]: df2
Out[33]:
a b c
0 0.4871 0.6371 0.5722
1 0.8823 0.8011 0.8852
2 0.9926 0.1276 0.0486
3 0.0866 0.5796 0.2961
.. ... ... ...
8 0.3562 0.7629 0.8319
9 0.4292 0.4811 0.6428
10 0.6572 0.5602 0.7924
11 0.1042 0.1500 0.3098
[12 rows x 3 columns]
In [34]: expr = '0.0 <= a <= c <= 0.5'
In [35]: map(lambda frame: frame.query(expr), [df, df2])
Out[35]:
[Empty DataFrame
Columns: [a, b, c]
Index: [], a b c
3 0.0866 0.5796 0.2961
11 0.1042 0.1500 0.3098]
2.14.3 query()
Python versus pandas Syntax Comparison
Full numpy-like syntax
In [36]: df = pd.DataFrame(np.random.randint(n, size=(n, 3)), columns=list('abc'))
In [37]: df
Out[37]:
a b c
0 0 8 9
1 3 3 9
2 4 1 6
3 6 9 4
.. .. .. ..
6 0 9 3
7 5 4 2
8 6 3 3
9 4 5 6
[10 rows x 3 columns]
In [38]: df.query('(a < b) & (b < c)')
Out[38]:
a b c
0 0 8 9
9 4 5 6
In [39]: df[(df.a < df.b) & (df.b < df.c)]
Out[39]:
a b c
0 0 8 9
9 4 5 6
Slightly nicer by removing the parentheses (by binding making comparison
operators bind tighter than &
/|
)
In [40]: df.query('a < b & b < c')
Out[40]:
a b c
0 0 8 9
9 4 5 6
Use English instead of symbols
In [41]: df.query('a < b and b < c')
Out[41]:
a b c
0 0 8 9
9 4 5 6
Pretty close to how you might write it on paper
In [42]: df.query('a < b < c')
Out[42]:
a b c
0 0 8 9
9 4 5 6
2.14.4 The in
and not in
operators
query()
also supports special use of Python’s in
and
not in
comparison operators, providing a succinct syntax for calling the
isin
method of a Series
or DataFrame
.
# get all rows where columns "a" and "b" have overlapping values
In [43]: df = pd.DataFrame({'a': list('aabbccddeeff'), 'b': list('aaaabbbbcccc'),
....: 'c': np.random.randint(5, size=12),
....: 'd': np.random.randint(9, size=12)})
....:
In [44]: df
Out[44]:
a b c d
0 a a 3 1
1 a a 0 3
2 b a 3 4
3 b a 0 3
.. .. .. .. ..
8 e c 1 0
9 e c 4 7
10 f c 0 1
11 f c 0 0
[12 rows x 4 columns]
In [45]: df.query('a in b')
Out[45]:
a b c d
0 a a 3 1
1 a a 0 3
2 b a 3 4
3 b a 0 3
4 c b 4 0
5 c b 4 7
# How you'd do it in pure Python
In [46]: df[df.a.isin(df.b)]
Out[46]:
a b c d
0 a a 3 1
1 a a 0 3
2 b a 3 4
3 b a 0 3
4 c b 4 0
5 c b 4 7
In [47]: df.query('a not in b')
Out[47]:
a b c d
6 d b 3 1
7 d b 1 2
8 e c 1 0
9 e c 4 7
10 f c 0 1
11 f c 0 0
# pure Python
In [48]: df[~df.a.isin(df.b)]
Out[48]:
a b c d
6 d b 3 1
7 d b 1 2
8 e c 1 0
9 e c 4 7
10 f c 0 1
11 f c 0 0
You can combine this with other expressions for very succinct queries:
# rows where cols a and b have overlapping values and col c's values are less than col d's
In [49]: df.query('a in b and c < d')
Out[49]:
a b c d
1 a a 0 3
2 b a 3 4
3 b a 0 3
5 c b 4 7
# pure Python
In [50]: df[df.b.isin(df.a) & (df.c < df.d)]
Out[50]:
a b c d
1 a a 0 3
2 b a 3 4
3 b a 0 3
5 c b 4 7
7 d b 1 2
9 e c 4 7
10 f c 0 1
Note
Note that in
and not in
are evaluated in Python, since numexpr
has no equivalent of this operation. However, only the in
/not in
expression itself is evaluated in vanilla Python. For example, in the
expression
df.query('a in b + c + d')
(b + c + d)
is evaluated by numexpr
and then the in
operation is evaluated in plain Python. In general, any operations that can
be evaluated using numexpr
will be.
2.14.5 Special use of the ==
operator with list
objects
Comparing a list
of values to a column using ==
/!=
works similarly
to in
/not in
In [51]: df.query('b == ["a", "b", "c"]')
Out[51]:
a b c d
0 a a 3 1
1 a a 0 3
2 b a 3 4
3 b a 0 3
.. .. .. .. ..
8 e c 1 0
9 e c 4 7
10 f c 0 1
11 f c 0 0
[12 rows x 4 columns]
# pure Python
In [52]: df[df.b.isin(["a", "b", "c"])]
Out[52]:
a b c d
0 a a 3 1
1 a a 0 3
2 b a 3 4
3 b a 0 3
.. .. .. .. ..
8 e c 1 0
9 e c 4 7
10 f c 0 1
11 f c 0 0
[12 rows x 4 columns]
In [53]: df.query('c == [1, 2]')
Out[53]:
a b c d
7 d b 1 2
8 e c 1 0
In [54]: df.query('c != [1, 2]')
Out[54]:
a b c d
0 a a 3 1
1 a a 0 3
2 b a 3 4
3 b a 0 3
.. .. .. .. ..
6 d b 3 1
9 e c 4 7
10 f c 0 1
11 f c 0 0
[10 rows x 4 columns]
# using in/not in
In [55]: df.query('[1, 2] in c')
Out[55]:
a b c d
7 d b 1 2
8 e c 1 0
In [56]: df.query('[1, 2] not in c')
Out[56]:
a b c d
0 a a 3 1
1 a a 0 3
2 b a 3 4
3 b a 0 3
.. .. .. .. ..
6 d b 3 1
9 e c 4 7
10 f c 0 1
11 f c 0 0
[10 rows x 4 columns]
# pure Python
In [57]: df[df.c.isin([1, 2])]
Out[57]:
a b c d
7 d b 1 2
8 e c 1 0
2.14.6 Boolean Operators
You can negate boolean expressions with the word not
or the ~
operator.
In [58]: df = pd.DataFrame(np.random.rand(n, 3), columns=list('abc'))
In [59]: df['bools'] = np.random.rand(len(df)) > 0.5
In [60]: df.query('~bools')
Out[60]:
a b c bools
4 0.9722 0.6328 0.7278 False
7 0.9512 0.4674 0.4987 False
In [61]: df.query('not bools')
Out[61]:
a b c bools
4 0.9722 0.6328 0.7278 False
7 0.9512 0.4674 0.4987 False
In [62]: df.query('not bools') == df[~df.bools]
Out[62]:
a b c bools
4 True True True True
7 True True True True
Of course, expressions can be arbitrarily complex too
# short query syntax
In [63]: shorter = df.query('a < b < c and (not bools) or bools > 2')
# equivalent in pure Python
In [64]: longer = df[(df.a < df.b) & (df.b < df.c) & (~df.bools) | (df.bools > 2)]
In [65]: shorter
Out[65]:
Empty DataFrame
Columns: [a, b, c, bools]
Index: []
In [66]: longer
Out[66]:
Empty DataFrame
Columns: [a, b, c, bools]
Index: []
In [67]: shorter == longer
Out[67]:
Empty DataFrame
Columns: [a, b, c, bools]
Index: []
2.14.7 Performance of query()
DataFrame.query()
using numexpr
is slightly faster than Python for
large frames
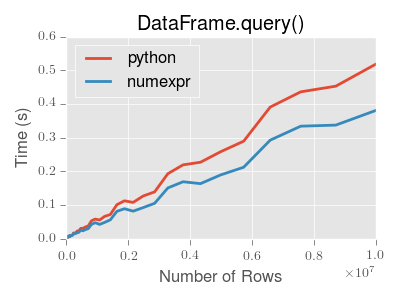
Note
You will only see the performance benefits of using the numexpr
engine
with DataFrame.query()
if your frame has more than approximately 200,000
rows
This plot was created using a DataFrame
with 3 columns each containing
floating point values generated using numpy.random.randn()
.